Deep learning is the new buzz-term in the tech world, defining a new level of machine learning that has captured the imaginations and pockets of some of the world’s biggest digital companies.
In the smart building and smart city sectors, deep learning is fostering the evolution of smarter sensors and reducing the overwhelming demands created by centralised big data. While the concept is not entirely new, recent activity in the market suggests that deep learning may represent a turning point in the machine intelligence story.
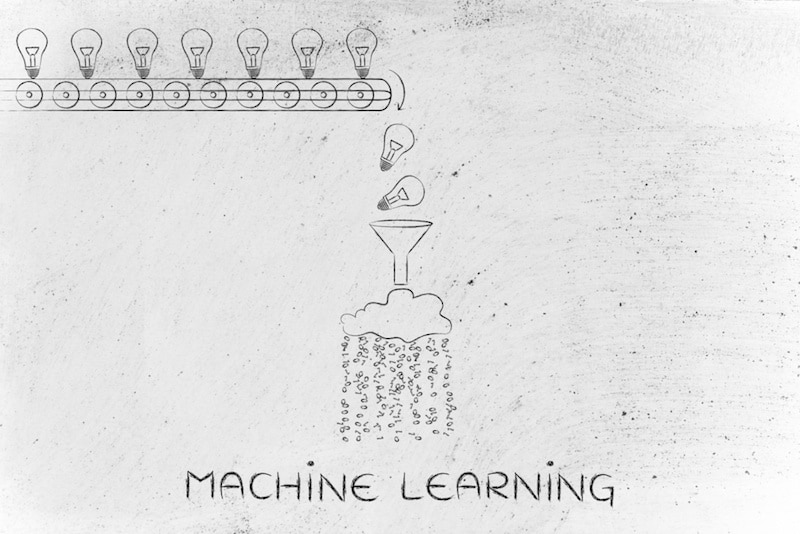
“Pattern recognition” is considered by many to be the birth of “smart” programming and was a term popular in the 70s and 80s. The emphasis was on getting a computer program to do something smart like recognise characters. In the 90’s came “Machine Learning”, which used algorithms to replace experts with data. With machine learning the emphasis was on the computer program (or machine) to do some learning after it is given data.
Machine Learning grew into a major research topic in the mid 2000s, computer scientists and tech corporations began applying these ideas to a wide array of problems. Machine Learning became an integral part of robotics, recognition software and was soon one of the most desired and versatile computing skills in the business.
Now, alongside the developments of the Internet of Things (IoT) and Big Data, we have moved into the era of deep learning. Demonstrated in this Google Trends graph showing web searches of the three terms since 2004.
The evolution to deep learning has been enabled, in large part, by access to faster computing and vast amounts of data, which is necessary for training. Deep Learning has successfully addressed many problems that machine learning struggled with within image classification, voice recognition, language translation and so on. In the case in Building Automation, Deep Learning tools have significantly improved the algorithm’s that can detect people and their exact location and movement in a room, for example.
“Whereas in the rule-based system world (pattern recognition and machine learning) the system engineer needed exhaustive information about the domain in order to build a good system, in the Deep Learning world this is no longer necessary”, explained Jonathan Laserson, PointGrab Machine Learning Expert, in a recent white paper. “In this era of the IoT where new kinds of data are becoming available at a rapid clip, Deep Learning allows us to faster iterate on new data sources and use them to our best advantage without requiring intimate knowledge of them”.
Deep learning ability has now become the target for the who’s who of Silicon Valley. Earlier this month customer success platform and world's leading CRM company, Salesforce, acquired deep learning start-up MetaMind in a move to integrate machine learning and smart capabilities into its cloud-powered software. Salesforce has a strong appetite for such smart technologies having recently acquired machine learning startup PredictionIO and smart calendar app Tempo AI.
Also this month, Amazon, the world’s largest internet retailer, acquired Artificial Intelligence-based deep learning start-up Orbeus Inc. Underlining the efforts made by Amazon to dig deeper into the world of smart software for its businesses in the cloud computing and connected devices spheres. Orbeus has carved its niche in image processing by using AI, based on its ReKognition API. This technology allows other software developers to add facial or object recognition to their own products.
While at Google and Facebook, deep learning technologies developed internally are already helping identify faces in photos and video, recognise spoken commands, and all manner of smart applications. Looking forward, deep learning can help give smart technology the power to not only perform tasks but also learn them on the fly. Some even believe it can eventually give machines something close to “common sense”, leading us to the perhaps obvious conclusion that deep learning may be the precursor to Artificial Intelligence (AI).
Last December, at the end of a weeklong AI conference in Montreal, Elon Musk, founder of electric car firm Tesla, and Sam Altman, president of famed tech incubator Y Combinator, unveiled their new artificial intelligence organisation. ‘OpenAI’ is a non-profit artificial intelligence research company. The goal “is to advance digital intelligence in the way that is most likely to benefit humanity as a whole, unconstrained by a need to generate financial return”, according to an official statement.
[contact-form-7 id="3204" title="memoori-newsletter"]
Musk and Altman have battled to keep their best AI researchers from the grasp of other big tech firms. The pair worries that “if people can build AI that can do great things, then they can build AI that can do awful things too”. Musk and Altman are not alone in their fear of robot overlords, Stephen Hawking has claimed that AI could spell the end of mankind. However, and perhaps counterintuitively, Musk and Altman think the best way to battle malicious use of AI is not to restrict access to it, but expand it.
The deep learning movement was born in Academia with Yoshua Bengio, Geoff Hinton and Yann LeCun. Hinton and LeCun may have left academia for Google and Facebook because of the enormous resources of these companies, but they remain intent on collaborating with other thinkers. “Deep learning research requires this free flow of ideas. When you do research in secret, you fall behind”, said LeCun.
In stark contrast to the Silicon Valley norm, these big companies now share a lot of their AI research. Just recently, Google open sourced the software engine that drives its neural networks. Altman and Musk aim to push the notion of openness further still, saying they don’t want one or two large corporations controlling the future of AI. The implications for smart buildings, smart cities and the world at large cannot yet be predicted, nor understated.